AI In Healthcare - Where It’s Performing Well and Where It’s Not
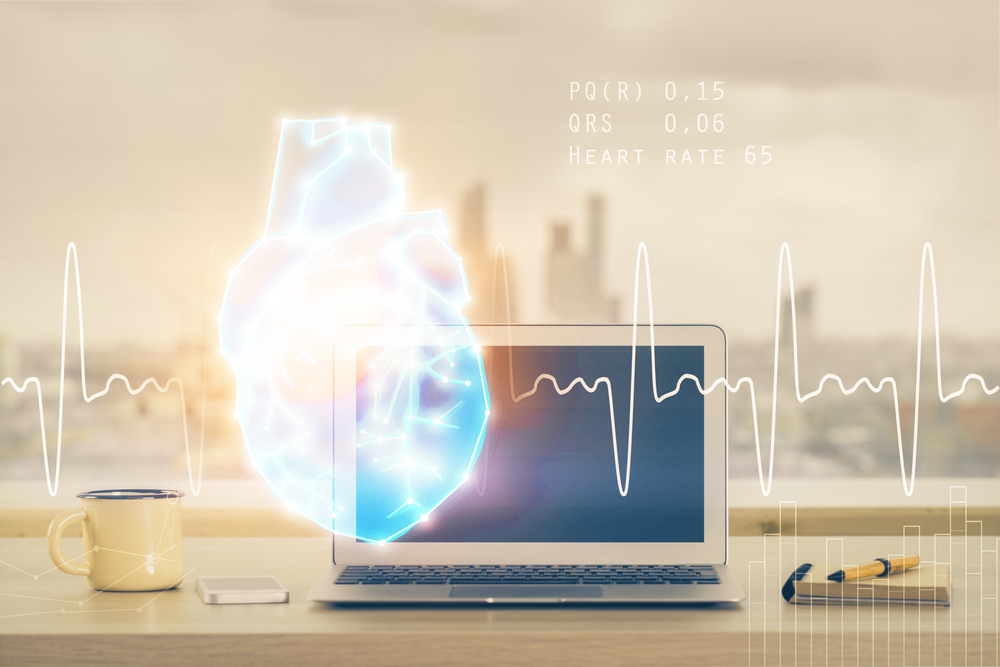
The future of AI in healthcare often conjures up images of robot doctors and virtual clinics. Certainly, recent breakthroughs in the field give reason to be optimistic, and market reports show a jump in healthcare AI investment from $2.6 billion to $4 billion between 2018 to 2019. But day-to-day usage of AI that dominates the field consists not of computerized medical staff but rather tools that cut down bureaucratic and administrative burden and aid in clinical decisions. According to a recent Center for Connected Medicine report, most survey respondents used AI tools for clinical decision support and dictation assistance or transcription. They’re certainly not the only AI tools in healthcare- medical imaging has become a strong area where AI has shown promise - but they highlight the areas where it’s performing well in addition to the expectations that haven’t quite been met yet, and why.
Where AI Has Performed Well
Improvements in NLP (natural language processing) have opened the door for better, more robust transcription tools that help physicians cut time on charting and documentation in their EHR. Companies like DeepScribe use AI-assisted scribes, and others like Nimblr offer virtual assistants that automate administrative tasks.
According to the Connected Medicine report, 48% of respondents also use AI tools for diagnostic medical imaging - the third highest use case for AI- and they’ve proved successful in several specialties. Ophthalmology providers have used computer vision systems that can predict whether a patient’s second eye will become higher risk for age-related macular degeneration, and companies such as Aidoc, Viz.ai, and Zebra Medical Vision are FDA-approved AI vendors who have undergone immense growth in the last couple of years as well.
Healthcare organizations have also been able to leverage AI in the treatment and/or administration of care for COVID-19 patients. 50% of respondents in the same survey reported using AI tools to help manage the COVID-19 crisis. A National Institute of Health literature review found that AI-based imaging tools were able to provide high degrees of accuracy in diagnosing COVID-19-induced pneumonia.
Harvard researchers also developed the COVID-19 Acuity Score (CoVA), a machine-learning model tool that predicts the prognosis of individuals who are likely to develop severe symptoms and require hospitalization.
In essence, transcription-related AI tools as well as those that assist providers in the clinical diagnostic process, particularly within medical imaging, have come a long way.
Where AI Hasn’t Delivered & Why
Despite the progress, hopes for diagnostic and clinical recommendations requiring little to no human assistance have not been realized quite yet. IBM’s Watson for Oncology was supposed to recommend cancer treatments, and while it’s still in use in some organizations, its expectations for groundbreaking work have not come to full fruition and has even faced criticism from the medical community.
One of the biggest reasons for these types of issues in the diagnostic realm is a lack of reliable and robust data required for building accurate algorithms and models. First, there’s the issue of accessing the data, which often lives in the EHR, and most EHR companies are not in the business of building diagnostic AI tools - although there is some evidence that these interoperability challenges are improving. Then there’s the hurdle of ensuring that the data being used is accurate and consistent. Most healthcare systems use less than 20% of their data for AI, and if AI tools are using models based on unclean data, a lot of incorrect and potentially harmful results could be realized.
“Big data analytics has potential, but healthcare data is very difficult to handle in comparison with business data,” says Yufei Yuan, Professor of Information Systems at McMaster University.
“Another issue is accountability. When using AI-based diagnoses, who will be responsible for mistakes? The designer or the user?”
Biases in data represent a third obstacle that often becomes entangled with ethical concerns, particularly facial recognition data which has been fraught with controversy in recent years. Facebook settled a class action lawsuit brought in 2015 that violated Illinois’ biometric privacy laws with their facial recognition data, and other states, such as Washington have either passed, or are beginning to introduce bills that curb facial recognition tools used by law enforcement. In the healthcare space, however, biases have different consequences. Basing models off of data from a particular population may lead to predictions that are not necessarily applicable to other populations who may have less access to healthcare services. If providers do not understand how their AI tools are coming to their conclusions, it’s hard to put full faith in their recommendations.
What’s Ahead
Not all facial recognition tools have harmful consequences though. NLP, facial recognition and computer vision systems have helped practitioners identify markers for certain mental health conditions and can support initial psychological screenings.
There’s also much optimism for AI-based medical imaging products. The Centers for Medicare and Medicaid (CMS) approved the first-ever reimbursement this year for a deep-learning based product by Viz.ai that uses a system to detect blockages in the brain. The FDA has also approved several AI-based diagnostic software in the last couple of years, such as IDx-DR for diabetic retinopathy detection and Imagen OsteoDetect software for wrist fracture detection.
Other developments on the horizon include AI-designed drugs. A clinical trial for a medication to treat OCD is underway in Japan, and graph neural networks have been tested out for new antibiotic compounds over the past year as well.
AI undoubtedly shows promise, and investment in the technology signals its potential. Roughly 500 AI startups raised over $8.4 billion of funding in Q1 of this year, and 44% of respondents in the Top of Mind survey reported increasing their AI investment as a result of the pandemic. Still, AI technology that drastically improves clinical outcomes leaves much to be desired.